Machine Learning in 2024: Trends and Predictions
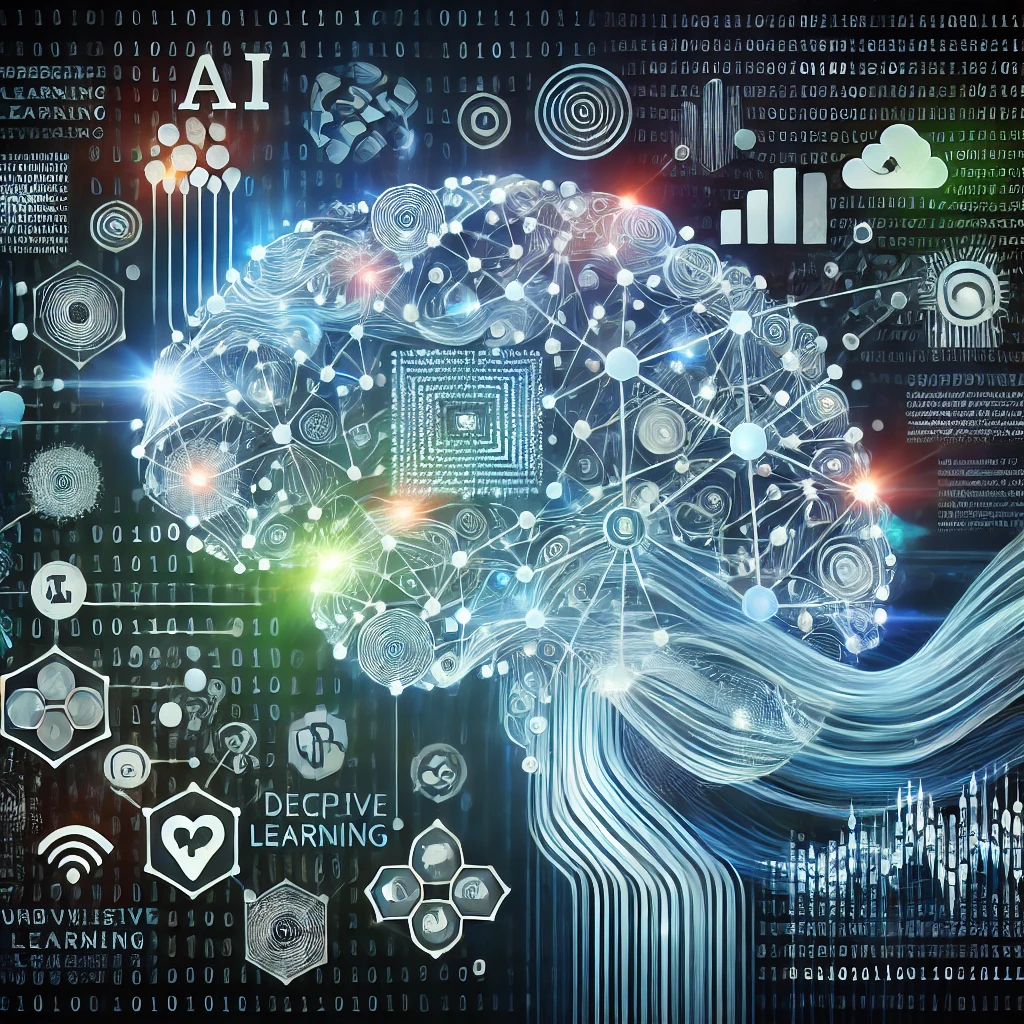
Categories:
8 minute read
Machine learning (ML) continues to be a driving force behind innovation in technology, with its influence expanding across industries such as healthcare, finance, manufacturing, and entertainment. As we move into 2024, machine learning is becoming more embedded in the fabric of everyday life and is poised to tackle even more complex challenges. The field is evolving at a rapid pace, with breakthroughs in algorithms, computing power, and data availability shaping its future. In this blog post, we’ll explore the trends that will define machine learning in 2024 and beyond, and predict where the technology is headed in the coming years.
The Growing Importance of Foundation Models
In 2024, the evolution of foundation models—large, pre-trained models that can be fine-tuned for specific tasks—is likely to be one of the most transformative trends in machine learning. These models, such as OpenAI’s GPT series or Google’s BERT, have significantly improved natural language understanding, image recognition, and even complex decision-making. Their versatility comes from the fact that they are pre-trained on enormous datasets and can then be adapted to various tasks with minimal additional training.
The next wave of foundation models is expected to push the boundaries even further. These models will be capable of handling multi-modal inputs, integrating text, images, audio, and possibly video into unified frameworks. This capability would open new avenues for applications in areas such as virtual assistants, autonomous vehicles, and medical diagnostics. With their capacity to generalize across domains, foundation models are likely to lead to more robust, scalable AI systems that can be applied to a broader range of industries with minimal customization.
Trend Toward Federated Learning and Data Privacy
As concerns over data privacy continue to grow, federated learning is emerging as a critical trend in 2024. Federated learning allows machine learning models to be trained across multiple decentralized devices or servers while keeping the data localized. Instead of sending data to a central server, federated learning algorithms send updates about the model, thereby protecting users’ sensitive information.
In the era of heightened privacy regulations such as the General Data Protection Regulation (GDPR) in Europe and California’s Consumer Privacy Act (CCPA), this decentralized approach to model training offers a significant advantage. Organizations can still leverage vast amounts of data to build powerful machine learning systems, but without violating user privacy.
The adoption of federated learning is expected to increase, particularly in industries like healthcare and finance, where privacy is paramount. In healthcare, for example, patient data remains sensitive, and federated learning can enable medical research using distributed datasets from multiple hospitals without compromising confidentiality. Similarly, financial institutions can train fraud detection models without exposing user data to unnecessary risks. By 2024, federated learning could become a key component in building privacy-preserving machine learning models across various domains.
Machine Learning for Automation and Augmented Intelligence
Automation is a key application of machine learning, and its role will continue to expand in 2024. ML models are now automating routine tasks in industries such as manufacturing, customer service, and logistics, helping businesses streamline operations and reduce costs. But while automation will be a major trend, we’re also seeing a shift toward augmented intelligence, where AI and machine learning technologies complement human decision-making rather than replace it.
Augmented intelligence helps humans by providing data-driven insights and suggestions that improve decision-making in complex scenarios. For example, in healthcare, machine learning algorithms can analyze medical images and suggest diagnoses, but the final decision is made by a human doctor. Similarly, in finance, AI can identify patterns and trends in vast datasets, providing analysts with actionable insights.
In 2024, more businesses are expected to adopt augmented intelligence systems, as these hybrid models strike a balance between leveraging AI’s efficiency and maintaining human oversight. This trend will accelerate as machine learning systems become more explainable, helping humans understand and trust the decisions suggested by algorithms.
Expansion of Explainable AI (XAI)
As machine learning models become more complex, particularly with deep learning techniques, the issue of transparency and explainability becomes more urgent. Many current ML systems, especially those using neural networks, operate as “black boxes,” where their internal workings are difficult for even their developers to understand. This lack of transparency can be problematic in industries like healthcare, finance, and autonomous driving, where understanding why a model made a certain decision is crucial.
In 2024, there is a growing push toward Explainable AI (XAI)—developing techniques that allow machine learning models to explain their decisions in a way that humans can understand. Techniques such as LIME (Local Interpretable Model-agnostic Explanations) and SHAP (SHapley Additive exPlanations) are gaining traction as tools that provide insights into how a model arrived at a particular conclusion.
The demand for XAI is being driven not just by regulatory concerns but also by the need to build trust in AI systems. As machine learning becomes embedded in critical decision-making processes, organizations must ensure that the technology is transparent, interpretable, and accountable. XAI will be a critical trend in 2024, particularly in industries where understanding the “why” behind a model’s decision is just as important as the “what.”
Democratization of Machine Learning Tools
Machine learning is becoming increasingly accessible, thanks to the democratization of tools and platforms that allow even non-experts to build and deploy models. In 2024, AutoML (Automated Machine Learning) and no-code/low-code platforms are expected to further break down the barriers to entry, enabling more individuals and small businesses to leverage machine learning in their operations.
AutoML platforms, such as Google’s Cloud AutoML and Microsoft’s Azure ML, automate many of the tasks involved in building machine learning models, such as data preprocessing, feature selection, and hyperparameter tuning. This allows users with little to no coding experience to develop sophisticated machine learning models that were once the domain of experts.
No-code and low-code platforms are also gaining momentum, allowing users to build custom AI applications with drag-and-drop interfaces. In 2024, these platforms will become even more user-friendly and powerful, enabling businesses of all sizes to incorporate AI into their workflows. The democratization of machine learning will drive greater innovation across industries, as more people gain access to the tools needed to harness the power of AI.
Real-Time Machine Learning and Edge AI
As demand for faster decision-making increases, real-time machine learning and Edge AI are poised to become even more critical in 2024. Real-time machine learning enables models to process and act on data as it is generated, without delays caused by batch processing. This is particularly important in use cases like fraud detection, recommendation systems, and autonomous vehicles, where decisions must be made instantly.
Edge AI takes real-time processing a step further by moving AI computation away from centralized cloud servers and closer to the data source, such as IoT devices, sensors, and mobile devices. By performing computations at the edge, these systems reduce latency, improve speed, and enhance privacy since data doesn’t need to be sent to a remote server for processing.
The rise of 5G networks will further accelerate the adoption of Edge AI in 2024, as faster network speeds will make it easier to deploy machine learning models on devices with limited computational resources. In industries like manufacturing, energy, and transportation, Edge AI will enable predictive maintenance, optimize energy consumption, and enhance the safety and efficiency of autonomous systems.
Predictive Analytics and AI-Driven Decision Making
Predictive analytics has been a cornerstone of machine learning for years, but in 2024, AI-driven decision-making is expected to take it to new heights. Machine learning models are now capable of analyzing vast amounts of historical data to predict future outcomes with increasing accuracy. This trend will continue to grow, with more organizations using predictive analytics to optimize operations, improve customer experiences, and gain a competitive edge.
In finance, for example, predictive models can forecast market trends, identify investment opportunities, and assess credit risk. In healthcare, machine learning models can predict disease outbreaks, patient readmissions, and treatment outcomes, helping providers make proactive decisions that improve patient care. Retailers are also using predictive analytics to forecast demand, personalize recommendations, and optimize inventory management.
As machine learning models become more sophisticated, the predictions they generate will be more accurate, reliable, and actionable. AI-driven decision-making will allow businesses to make data-backed decisions more quickly and confidently, leading to better outcomes across industries.
Conclusion: What to Expect in 2024 and Beyond
The landscape of machine learning in 2024 is marked by significant advancements in both technology and application. Foundation models will become more powerful and versatile, federated learning will provide privacy-conscious approaches to data sharing, and automation will continue to evolve into augmented intelligence, enhancing rather than replacing human decision-making. Explainable AI will play a vital role in building trust, while AutoML and no-code platforms will democratize access to machine learning tools. Real-time machine learning and Edge AI will meet the growing demand for immediate, decentralized decision-making, while predictive analytics will drive AI-powered insights across industries.
The year 2024 promises exciting developments in machine learning, with innovation continuing at a rapid pace. As these trends unfold, machine learning will become more integrated into our daily lives, shaping the future in ways we are only beginning to imagine. Organizations that embrace these trends will be well-positioned to lead in this new era of intelligent systems, while those that lag behind may struggle to keep up with the transformative potential of machine learning.
Feedback
Was this page helpful?
Glad to hear it! Please tell us how we can improve.
Sorry to hear that. Please tell us how we can improve.